- Published on
Regional indonesian language translator
Machine Translation (MT) for Balinese: out of reach?
Four years in Timor-Leste and pandemic lockdowns gave me ample time and incentives to develop tetun.org, a machine translation service for the Tetun language. After I moved to Indonesia in 2020, I became more and more interested in its regional languages, Balinese in particular.
Machine translation systems rely on large amounts of written text to train themselves. Since I could find significantly less text for Balinese than I did for Tetun, my hopes for creating a machine translation system for the language were initially low. However, fast advances in low-resource machine translation, in particular by the Masakhane community leveraging existing multilingual models, raised my hopes that machine translation for Balinese was within reach.
NLLB and its many regional Indonesian languages
It was with some surprise though that I read that Meta AI’s latest translation model, NLLB, not only covered Balinese, but also several other regional Indonesian languages that have no widely-used translation systems, namely Acehnese, Banjarese, Buginese, and the Minangkabau language. This came on top of better machine translation quality for Javanese and Sundanese than Google Translate!
So after trying out NLLB, and getting some motivation from my friend Joseagush to look further into it, I set out to put together a website that would leverage NLLB for regional Indonesian languages, alongside English and Indonesian. We published it on Twitter and it kinda blew up!
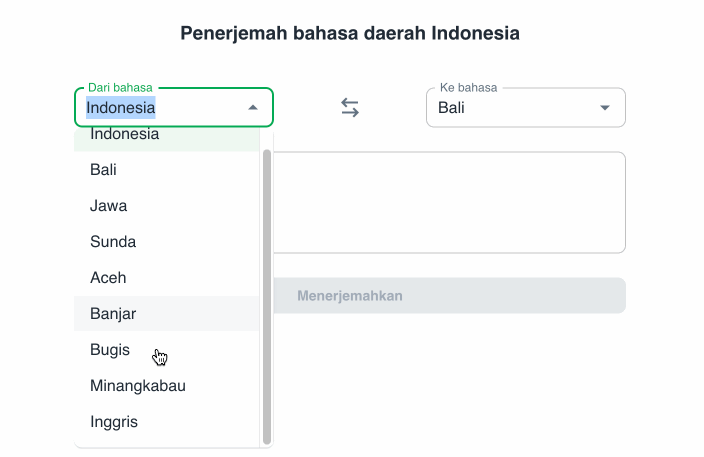
Looking ahead
The interest this generated motivates me to develop it. There’s no shortage of potential improvements:
- Improve translation quality for existing languages, especially through finding text covering different domains (news, educational content, everyday conversations)…
- … and better handle different registers for each language -- see this thread on Sundanese
- Add more languages, in particular there is hope for Madurese, Ngaju and Batak
- Polish the website design, this is still a prototype!
- Add dictionary entries, with examples for each of them, like we do on tetun.org
- And one day produce a mobile app…
- which ideally would work offline, so people in remote areas can translate without having internet access
There’s plenty of work ahead, but each task is fascinating in itself.
Improvements through symbiosis
It’s worth acknowledging here how valuable text from Wikipedia is when training MT systems. Thanks to its (high-quality content that covers many different domains (history, science, politics, etc), Wikipedia is a gold mine for finding training data. Similarly, once an MT system has been setup for a language, it can be used by Wikipedia editors to assist them in creating new articles from translations.
Overall, Indonesia is a fascinating country for machine translation, because even though its languages range from high-resource (Indonesian, and to a lesser extent Javanese) to low-resource, the close relationship between different languages means that a multilingual system learns “closely related” languages together. For example, a system that already knows Javanese will need less text to learn Balinese than a system that is trained exclusively on Balinese text. This is useful technologically, and in my opinion, a beautiful symbol for the country.